Short summary of my experience at ProbAI 2019
Brief intro
I attended a summer school about probabilistic machine learning in the first week of June this year in 2019. Probabilistic machine learning (sometimes Bayesian Machine Learning) is a discipline of machine learning where the uncertainty of a decision is also calculated. This is really interesting topic for many medical and other mission critical applied research now, as often a yes-or-no decision is not enough.
The venue
Trondheim is absoutely stunning, but not cheap. If you are looking for some untouched nature or a retreat from your PhD life, I definitely recommend it, but be wary - apart from nature there is not too much to see! However, all the restaurants were top notch in Trondheim. I would definitely recommend Peppes Pizza if you are into greasy Domino’s like Pizza.
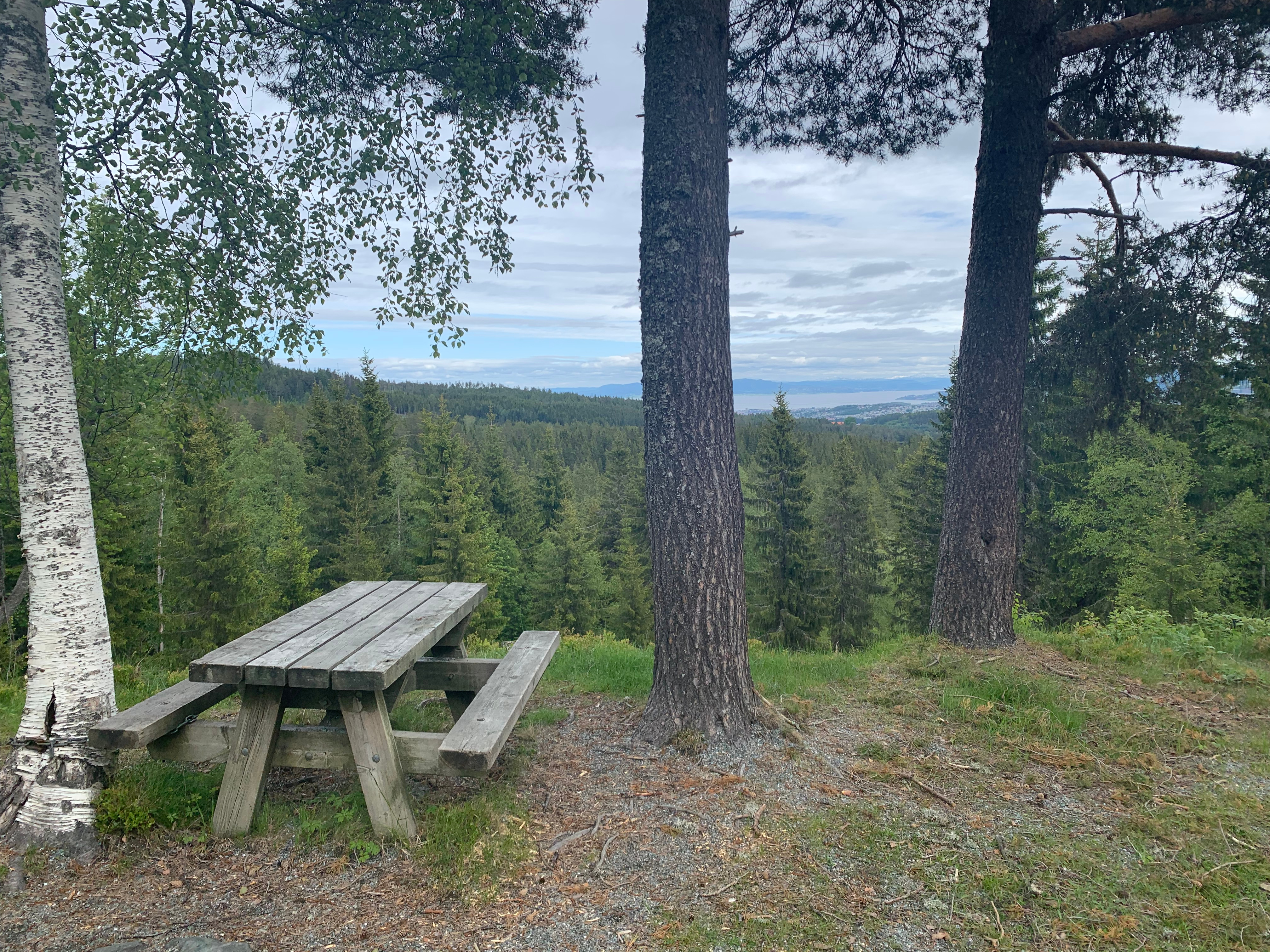
A bird's eye view of Trondheim
Lectures I liked the most
The two talks that I definitely adored the most were the one about Importance Weighted Autoencoders and the one about Sparse Bayesian Neural Networks. I have done my Master’s thesis about automatic relevance determination, and I think it’s one of the most appealing way to do Bayesian for people, who are looking for a principled framework, dealing with prior probability distributions.
What I’ve learned
I think it was a great practical look at Bayesian Machine Learning and Probabilistic Graphical models. It was refreshing to have a great review of the past few years machine learning literature, and I got confirmed that cycle-consistent GANs (and GANS in general) are indeed becoming more than a niche ML research area.
The related coursework was one of the most rewarding assignments I have ever done, because it really helped me bring together the theory and practice in my mind.
Depending on next year’s topics I might consider attending again, but it was definitely a refreshing breakout from my PhD life.
This project has received funding from the European Union’s Horizon 2020 research and innovation programme under Marie Sklodowska-Curie grant agreement No 766287.